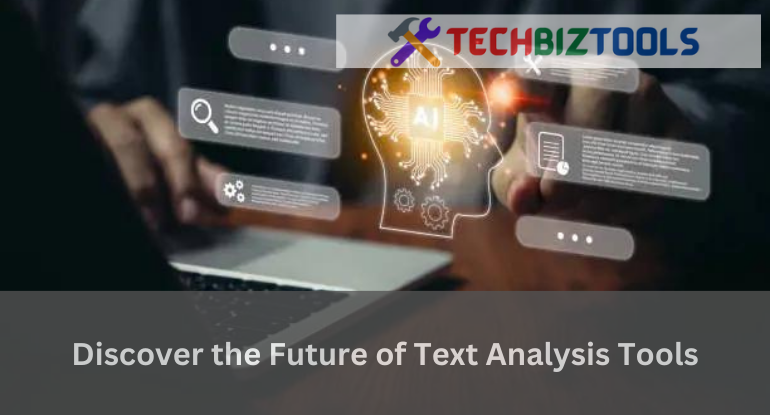
Discover the Future of Text Analysis Tools
Text analysis tools have revolutionized how we process written content. These tools have moved to understand context, sentiment, and intent, aiding businesses, researchers, and individuals in extracting insights from vast textual data.
Text analysis is increasingly vital in our information-rich world, enabling data-driven decisions, scholarly research, and language appreciation.
Its relevance extends to various domains like sentiment analysis and chatbots, as these tools continue to advance in their capabilities.
This comprehensive article will look into the latest developments in English text analysis, showcasing real-world applications and the evolving role of these tools in our daily lives.
Evolution of Text Analysis
Text analysis has come a long way over the years, and it continues to evolve at a remarkable pace. The tools and techniques used to analyze written language have undergone significant advancements, enabling us to gain deeper insights into the meaning, sentiment, and structure of texts.
This evolution has been driven by various factors, including advancements in natural language processing (NLP), machine learning, and artificial intelligence (AI).
One of the most noteworthy trends in text analysis is the increasing use of machine learning models like deep learning and transformer models.
These models, such as BERT and GPT-3, have the ability to understand context and contextually analyze text, making them invaluable for tasks like sentiment analysis, entity recognition, and summarization.
This has opened up new possibilities in the field of content analysis, as these models can now generate more accurate and contextually relevant insights from vast amounts of text data.
Additionally, the integration of text analysis into various industries has been on the rise. From marketing and customer support to healthcare and finance, organizations are leveraging text analysis tools to extract actionable insights from unstructured text data.
For example, businesses are using sentiment analysis to gauge customer feedback and adjust their strategies accordingly, while healthcare professionals are utilizing text analysis to mine medical records for valuable information.
Another exciting development is the increasing focus on multilingual text analysis. With the global nature of the internet, the ability to analyze text in multiple languages is becoming essential.
Advances in cross-lingual models and the availability of multilingual training data are making it easier to perform accurate and reliable text analysis in a variety of languages, breaking down language barriers and broadening the scope of content analysis.
Furthermore, the use of text analysis in social media monitoring and real-time data analysis is becoming more prevalent. This trend enables organizations and individuals to stay up to date with the latest trends, public sentiment, and breaking news.
By harnessing the power of text analysis in real-time, decision-makers can make more informed choices and react swiftly to changing circumstances.
The Role of Natural Language Processing (NLP)
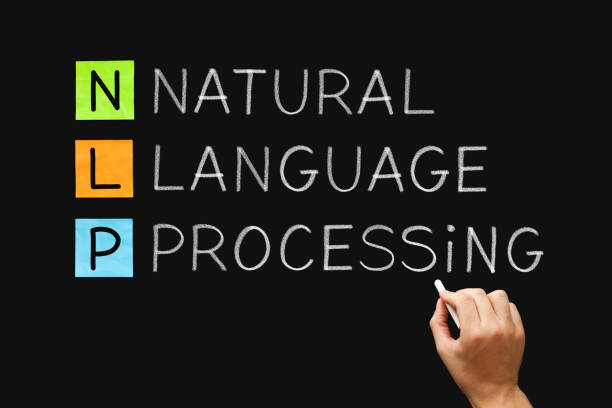
Natural Language Processing (NLP) is not merely a supporting actor in the area of text analysis; it's a star player that is transforming the aspect of English language analysis.
NLP has emerged as a powerhouse technology that has been instrumental in redefining the way we interact with and understand human language.
It is the intersection of linguistics and computer science, aiming to enable machines to comprehend, interpret, and generate human language. The applications of NLP are incredibly diverse and extend to nearly every facet of our lives.
One of the key ways NLP is influencing the future of English language analysis is through its ability to facilitate the extraction of insights from vast amounts of unstructured text data.
This encompasses everything from social media posts and news articles to customer reviews and medical records. With NLP, we can identify sentiment, extract key information, and even generate concise summaries, which are pivotal for decision-making, research, and understanding public opinion.
The impact of NLP on industries is undeniable. In the aspect of marketing and customer service, companies are using NLP-powered chatbots and sentiment analysis to engage with customers and gain deeper insights into their preferences and needs.
In healthcare, NLP is enhancing the analysis of medical records and aiding in diagnosing diseases, thereby improving patient care and research. Additionally, the finance sector is using NLP for sentiment analysis of market news and customer feedback to make informed investment decisions.
These applications not only increase efficiency but also lead to more customer-centric and data-driven approaches.
In daily life, NLP is becoming increasingly integrated. Virtual assistants like Siri, Alexa, and Google Assistant rely heavily on NLP to understand and respond to spoken commands and queries.
This technology allows us to interact with our devices in a more natural and intuitive manner, simplifying tasks such as setting reminders, searching for information, or controlling smart home devices.
Furthermore, NLP is a driving force behind the development of multilingual capabilities in text analysis. As the world becomes more interconnected, the need to analyze text in various languages is growing.
NLP models that can understand and work with multiple languages are bridging language barriers, making global communication and data analysis more accessible and effective.
Machine Learning in Text Analysis
Machine learning, a cornerstone of modern technological innovation, lies at the core of text analysis tools.
Machine learning, a subfield of artificial intelligence, has revolutionized the way we process and make sense of vast volumes of textual data. It equips computers with the ability to learn and adapt from data, allowing them to autonomously improve their performance and make predictions.
In the context of text analysis, machine learning acts as a catalyst, enabling us to decipher complex patterns, sentiments, and insights hidden within the vast sea of written language.
The applications of machine learning in text analysis are virtually boundless. One of its primary utilities is sentiment analysis, where it can automatically gauge the emotional tone of text, helping businesses understand customer feedback, evaluate product reviews, and make data-driven decisions to enhance their offerings.
This is just one example of how machine learning empowers organizations to connect with their audience and fine-tune their strategies.
Moreover, machine learning is the driving force behind entity recognition, a crucial component of text analysis. This technology enables systems to identify and classify entities like names of people, organizations, and locations within texts.
This is invaluable in various sectors, from news organizations that want to automatically tag relevant stories to healthcare institutions that need to extract vital patient information from medical records.
One of the pivotal advancements in machine learning for text analysis is the emergence of deep learning models. Transformers, such as BERT and GPT-3, have redefined the way we process and generate human language.
These models can understand context, enabling more accurate and contextually relevant analysis. They have found applications in tasks like text summarization, language translation, and content generation, creating new opportunities for content creators and researchers alike.
Machine learning has also fostered advancements in multilingual text analysis. In an increasingly globalized world, being able to analyze text in multiple languages is a game-changer.
Cross-lingual models, often powered by machine learning, bridge language gaps, making it possible to analyze text data from various linguistic backgrounds and opening up a world of possibilities for cross-cultural communication and insights.
Future Predictions
The future of text analysis holds the promise of transformative impacts across various sectors. One of the most exciting prospects is the advancement of artificial intelligence (AI) models that can not only analyze text but also generate it in a human-like manner.
This evolution opens the door to highly personalized content creation, where AI-driven systems can craft engaging stories, marketing materials, or even legal documents, tailored to the unique preferences and needs of the audience.
Furthermore, the integration of text analysis into everyday life will likely become even more seamless. We can anticipate an expansion of AI-driven virtual assistants that can not only respond to our spoken or written commands but also anticipate our needs and provide context-aware information.
From suggesting personalized book recommendations based on our reading history to offering real-time language translation during global conversations, these assistants will enhance our daily communication and decision-making processes.
In the business world, text analysis is set to revolutionize market research. Advanced sentiment analysis tools will not only detect positive or negative sentiment but also understand the nuances and cultural context of language, providing companies with unparalleled insights into consumer opinions and trends. This will enable businesses to adapt their products and strategies with a higher degree of accuracy and responsiveness.
In healthcare, text analysis is poised to play a pivotal role in diagnostic and treatment processes. With the increasing volume of medical literature and patient records, AI-powered systems can assist doctors in staying up to date with the latest research and help identify potential health issues earlier by analyzing patients' medical history and symptoms. This could lead to more accurate diagnoses and improved patient care.
Moreover, the application of text analysis in content curation and recommendation systems will continue to evolve. As algorithms become more refined and personalized, we can expect to see more accurate news suggestions, product recommendations, and educational content tailored to our individual interests and preferences, contributing to a more informed and enriched digital experience.
The influence of text analysis will also extend to the legal and regulatory sectors, where AI tools can assist in reviewing contracts and legal documents, identifying potential risks and inconsistencies. This has the potential to streamline legal processes and reduce the risk of errors, making the legal system more efficient and accessible.
Implementing Text Analysis Tools
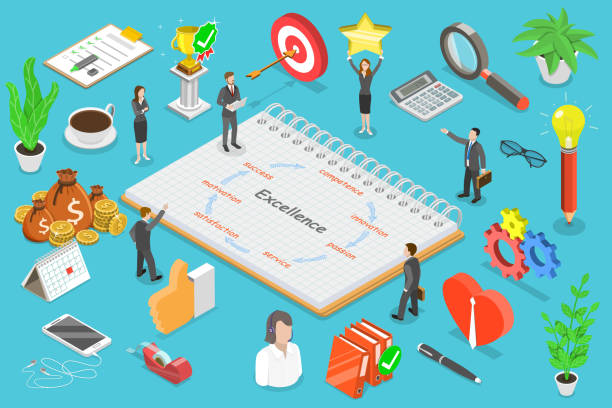
Embarking on the journey to implement text analysis tools for English content can be a transformative step in harnessing the power of language for various applications.
In this step-by-step guide, we will demystify the process and provide practical insights to help you successfully set up text analysis tools. Along the way, we'll recommend tools and strategies that can enhance your text analysis journey.
Step 1:
Define Your Objectives Begin by clearly outlining your goals and objectives. What do you aim to achieve with text analysis?
Are you interested in sentiment analysis, content categorization, or language translation?
Defining your purpose will guide the choice of tools and techniques you'll need.
Step 2: Select the Right Text Analysis Tools The choice of text analysis tools is critical. There are numerous options available, ranging from open-source libraries like NLTK and spaCy to cloud-based services such as Google Cloud Natural Language and IBM Watson NLU. Consider factors like cost, scalability, and ease of integration when making your selection.
Step 3: Gather and Prepare Data Data is the lifeblood of text analysis. Collect relevant text data that aligns with your objectives. Ensure it is clean and well-structured, as the quality of your input data directly impacts the accuracy of your analysis.
Step 4: Preprocessing Text Data Before analysis, you'll need to preprocess the text data. This involves tasks like tokenization, stemming or lemmatization, and removing stop words. Preprocessing ensures that your data is in a format suitable for analysis.
Step 5: Perform Text Analysis Apply the chosen text analysis tools to your preprocessed data. The specific techniques will vary based on your objectives.
For sentiment analysis, you'll classify text as positive, negative, or neutral. For topic modeling, you may use techniques like Latent Dirichlet Allocation (LDA) to extract topics from the text.
Step 6: Validate and Evaluate Results After analysis, it's crucial to validate and evaluate the results. Ensure that the insights generated align with your objectives. Assess the accuracy and performance of your analysis to identify areas for improvement.
Step 7: Iterative Refinement Text analysis is an iterative process. Use the insights gained from validation to refine your analysis. This may involve adjusting your tools, fine-tuning parameters, or acquiring additional training data to enhance accuracy.
Step 8: Integration and Deployment Integrate your text analysis tools into your workflow or application. Ensure that the analysis is seamlessly integrated with your existing systems and processes to make it a valuable part of your operations.
Step 9: Maintain and Update Text analysis tools and techniques evolve. Regularly update your tools and models to stay current with the latest advancements. Also, keep refining your analysis methods to adapt to changing requirements.
Step 10: Monitor and Optimize Continuously monitor the performance of your text analysis tools. Optimize for efficiency and accuracy. This may involve implementing automated alerts for specific insights or enhancing real-time analysis capabilities.
Conclusion
Text analysis tools revolutionize understanding, vital in business, healthcare, and research. Future promises AI-driven, personalized content, seamless virtual assistants, and enhanced analysis across industries.
Implementation involves defined objectives, tool selection, data preprocessing, iterative refinement, and continuous optimization.